Unlocking Success: The Role of Data Labeling in Machine Learning for Home Services
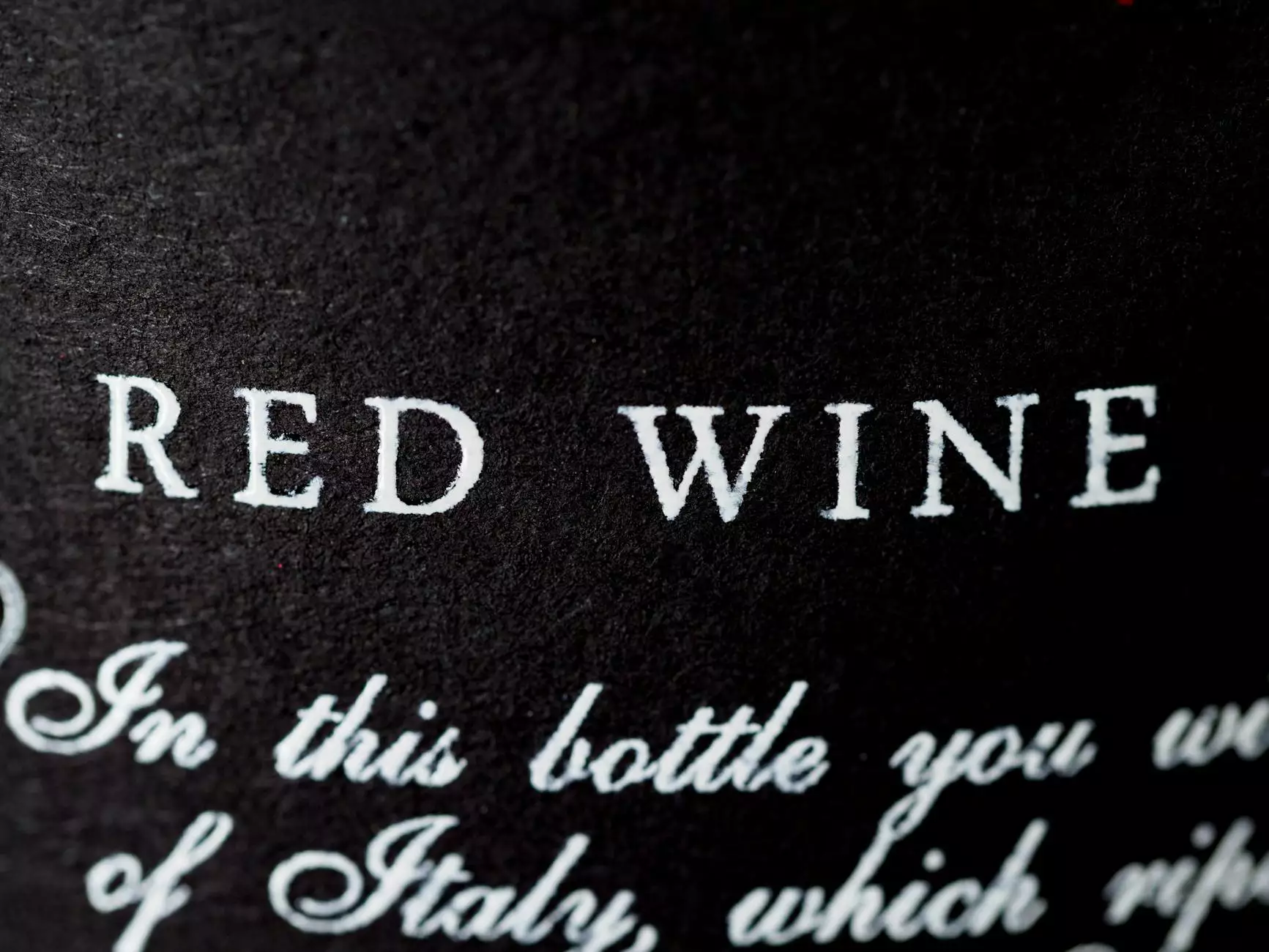
Within the ever-evolving realm of technology, data labeling has emerged as a pivotal element in driving the effectiveness of machine learning. In industries such as Home Services, especially in Keys & Locksmiths, the understanding and implementation of data labeling can significantly enhance operational efficiency and service delivery. In this article, we will delve into the intricate dynamics of data labeling in machine learning, its applications in the home services sector, and the underlying benefits that propel businesses like keymakr.com to achieve success.
The Foundation of Data Labeling in Machine Learning
Data labeling is the process wherein raw data is annotated with relevant information to enhance the learning capability of machine learning models. This transformative step is crucial for training algorithms to recognize patterns, predict outcomes, and ultimately make informed decisions. In a business landscape that heavily relies on data, understanding the nuances of data labeling can set an organization apart.
What is Data Labeling?
In simple terms, data labeling involves identifying and tagging data points in a dataset. This can include images, video footage, text, or even audio. The objective is to create a comprehensive dataset that machine learning models can learn from. For instance, in the context of locksmith services, data labeling could involve tagging images of various types of locks or categorizing service requests based on urgency.
Why is Data Labeling Important?
For machine learning models to be effective, they need high-quality, well-labeled data. The accuracy and reliability of predictions made by these models depend significantly on the quality of the training data. Here are several key reasons why data labeling is essential:
- Model Accuracy: Correctly labeled data ensures that the machine learning models can make accurate predictions, leading to better decision-making.
- Pattern Recognition: Enhanced pattern recognition capabilities enable businesses to understand customer behavior and service trends.
- Risk Mitigation: Accurate data labeling helps in identifying potential security risks more effectively, especially for locksmith services.
- Competitive Advantage: Businesses that invest in quality data labeling are better positioned to leverage insights that drive operational efficiencies.
Applications of Data Labeling in Home Services
The Home Services industry, particularly in the locksmith sector, can leverage data labeling in numerous ways. Here are some applications that demonstrate its significance:
1. Intelligent Customer Support Systems
By utilizing labeled data from previous customer interactions, machine learning models can be trained to deliver more effective customer support. Automated chatbots can understand and respond to customer inquiries accurately, thereby improving overall customer satisfaction.
2. Predictive Maintenance and Service Delivery
When data from services performed is labeled and analyzed, businesses can convert it into actionable insights. For instance, by understanding the frequency and type of lock issues reported by customers, a locksmith service can implement predictive maintenance schedules that minimize downtime and enhance service delivery.
3. Enhanced Marketing Strategies
Data labeling allows businesses to segment their customer base effectively. By categorizing clients based on their needs, preferences, and behaviors, locksmith services can tailor marketing campaigns that resonate more profoundly with their target audiences.
4. Fraud Detection and Security Measures
The application of machine learning in identifying fraudulent behaviors relies heavily on data labeling. Training models to recognize patterns associated with fraudulent activities helps locksmith services to mitigate risks and protect their customers.
The Process of Data Labeling
The execution of effective data labeling for machine learning involves a systematic approach. Here is a breakdown of the process:
1. Data Collection
The first step is to collect relevant data from various sources, such as customer service interactions, social media, operational logs, and more. The goal is to amass a diverse dataset that truly represents the operational landscape.
2. Annotation
Once the data is collected, the next step is annotation, where experts label the data according to predefined criteria. For instance, images of locks may be tagged with the type, brand, or specific features.
3. Quality Assurance
Quality assurance is crucial in ensuring that the labeled data is accurate and consistent. Implementing checks and balances during this phase can help identify and rectify errors, which is vital for maintaining the integrity of the dataset.
4. Model Training
With high-quality labeled data, the next step is to train the machine learning models. During this phase, the algorithms learn to identify patterns and make predictions based on the labeled data provided.
5. Continuous Improvement
Post-deployment, it is essential to continuously monitor the performance of the models and update them with new labeled data to maintain their accuracy and relevance.
Challenges in Data Labeling
Despite its advantages, data labeling presents several challenges that businesses in the Home Services sector must navigate:
1. Resource Intensive
Data labeling can be a time-consuming and costly process, requiring skilled personnel to ensure accuracy and quality, thereby straining resources.
2. Subjectivity
Different annotators may interpret data differently, leading to inconsistencies. Establishing clear guidelines is essential to mitigate this issue.
3. Scalability
As businesses expand, the volume of data grows exponentially, making it increasingly challenging to maintain consistent labeling practices while scaling operations.
Best Practices for Effective Data Labeling
To overcome the challenges of data labeling and harness its benefits, businesses should consider implementing the following best practices:
- Standardize Processes: Develop clear guidelines for data labeling to ensure consistent interpretations across various annotators and data types.
- Invest in Training: Ensure that team members responsible for data labeling are adequately trained to minimize subjectivity and errors.
- Utilize Automation: Where possible, leverage automated tools and software for labeling; this can significantly reduce time and increase productivity.
- Regular Audits: Conduct regular audits of the labeled data to identify any inaccuracies or inconsistencies and correct them promptly.
The Future of Data Labeling in Machine Learning
The landscape of machine learning and, by extension, data labeling is continuously evolving. Innovations are paving the way for greater efficiencies and more sophisticated algorithms. Here are some trends that may emerge:
1. Increased Use of AI and Automation
As technology advances, the role of artificial intelligence in automating the data labeling process is likely to expand, helping to reduce costs and improve efficiency.
2. Enhanced Collaboration Tools
New collaborative platforms designed for data labeling can allow teams to work seamlessly, facilitating faster and more accurate labeling while maintaining data integrity.
3. Emphasis on Ethical Considerations
As machine learning models are increasingly integrated into daily operations, the ethical implications of data usage and labeling are coming to the forefront. Emphasizing ethical data practices will be paramount in the years to come.
Conclusion
In conclusion, data labeling in machine learning is an indispensable aspect of the home services industry, particularly for businesses like keymakr.com. The investment in quality data labeling not only enhances operational performance but also drives significant improvements in customer satisfaction, security, and overall business success. By embracing best practices and staying abreast of industry trends, locksmith services and home service providers can unlock unprecedented opportunities for growth and innovation.
The journey of integrating data labeling and machine learning into business operations may be challenging, but the potential rewards greatly outweigh the challenges. With the right approach and resources, businesses can transform their data into actionable insights that lead to strategic advantage and sustainable success.
data labeling machine learning