The Transformative Power of Medical Datasets for Machine Learning
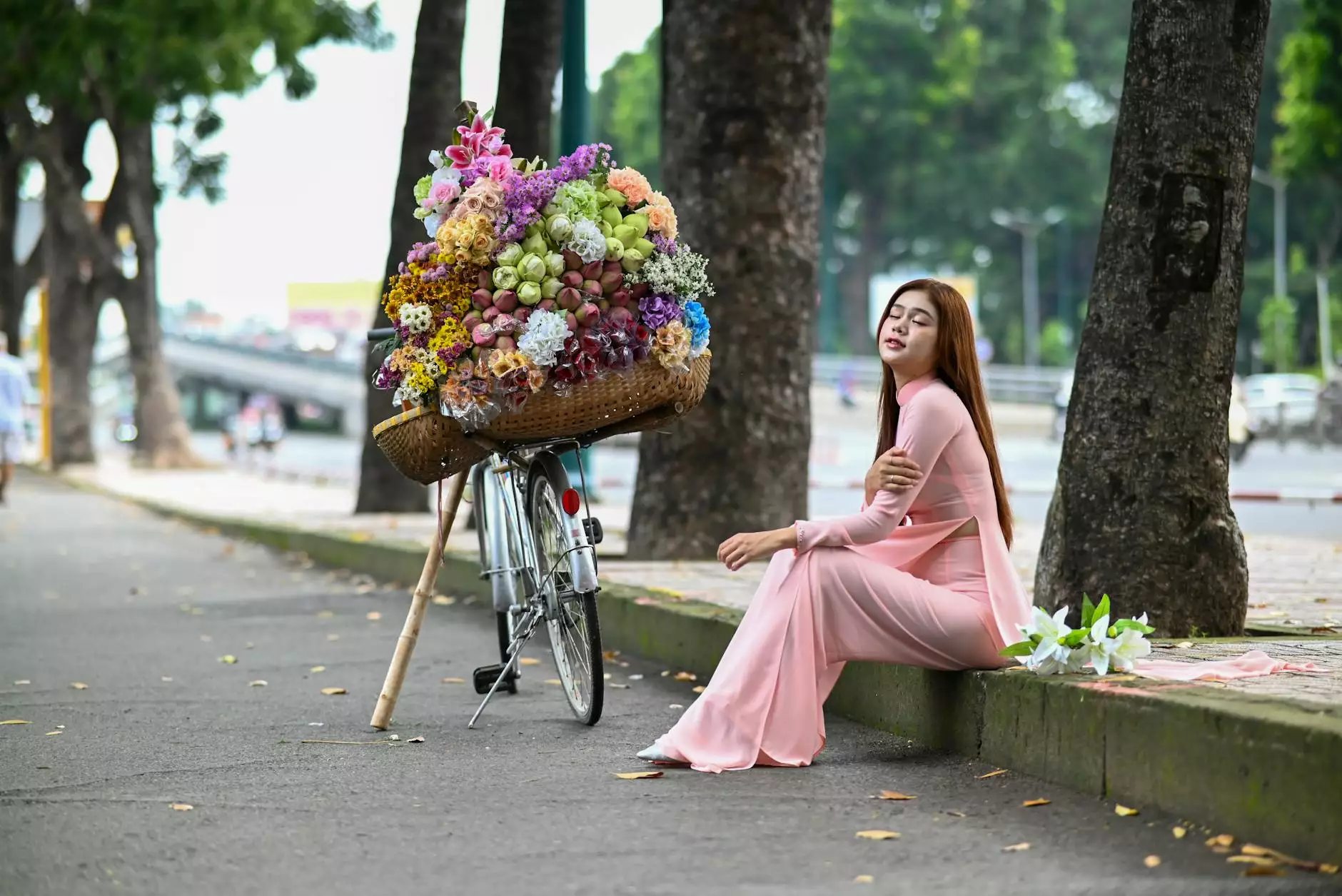
In today's rapidly evolving healthcare landscape, the integration of machine learning technology has become paramount. One of the cornerstones of this technological advancement is the accessibility and utilization of medical datasets for machine learning. These datasets are invaluable resources that hold the potential to drastically improve patient care and operational efficiencies within medical institutions.
Understanding Medical Datasets
Medical datasets consist of robust collections of health-related information that can be utilized for various research purposes. They encompass a vast range of data types, including:
- Electronic Health Records (EHRs): Comprehensive patient data, including demographics, medical history, and treatment plans.
- Clinical Trial Data: Information gathered during medical trials, providing insights into drug efficacy and patient responses.
- Genomic Datasets: Genetic information that fuels personalized medicine and targeted therapies.
- Radiology Images: Medical imaging data such as X-rays, MRIs, and CT scans, essential for diagnostic algorithm training.
The Critical Role of Machine Learning in Healthcare
Machine learning (ML) represents a subset of artificial intelligence (AI) that facilitates the processing and analysis of vast quantities of data. In healthcare, it serves several critical functions:
- Predictive Analytics: ML algorithms analyze historical patient data to predict future health outcomes, thereby enabling proactive care.
- Personalized Treatment Plans: By studying individual patient data, ML systems can recommend tailored treatment paths based on previous successful outcomes.
- Operational Efficiency: ML can streamline the processes within healthcare organizations, such as scheduling, resource allocation, and patient flow management.
- Early Detection of Diseases: Advanced models can identify subtle patterns in patient data that suggest the early presence of conditions such as cancer or heart disease.
Challenges in Obtaining Medical Datasets
Despite the potential advantages of utilizing medical datasets for machine learning, there are several challenges to be aware of:
- Data Privacy and Security: Patient data is sensitive, and strict regulations like HIPAA in the United States impose significant restrictions on data handling.
- Data Quality: Inaccurate or incomplete data can lead to erroneous conclusions, making data cleaning and validation crucial steps in the process.
- Data Integration: Merging datasets from different sources (hospitals, clinics, research institutions) often proves difficult due to varying formats and standards.
Gathering High-Quality Medical Datasets
For businesses and researchers keen on harnessing the power of machine learning, acquiring high-quality medical datasets is essential. Here are some effective strategies for obtaining these vital resources:
1. Collaborations with Healthcare Institutions
Partnering with hospitals and healthcare organizations can lead to valuable access to medical datasets for machine learning that are rich in structure and depth. Such collaborations not only facilitate data sharing but also ensure that ethical standards are met.
2. Publicly Available Datasets
Numerous governments and organizations provide open access to anonymized medical datasets. These sources are a goldmine for researchers looking to train machine learning models. Examples include:
- The National Institutes of Health (NIH) - offers datasets across various medical fields.
- PhysioNet - provides access to cardiovascular data and other critical health datasets.
- CDC Open Data - offers a range of health-related datasets for public use.
3. Synthetic Data Generation
In situations where using real patient data is impractical due to privacy concerns, synthetic datasets can be generated. These datasets mimic real-world data patterns and can be used to develop and test machine learning algorithms without risking patient confidentiality.
Leveraging Machine Learning on Medical Datasets
Once quality datasets are obtained, the journey of extracting insights through machine learning can begin. Here are several applications of machine learning that can be implemented:
1. Predictive Modeling
Predictive modeling uses historical data to forecast future events. In healthcare, this can mean predicting disease outbreaks based on infection rates, patient demographics, and environmental factors.
2. Natural Language Processing (NLP)
NLP techniques can analyze unstructured data from clinical narratives, helping to extract meaningful information that can contribute to patient diagnoses and treatment plans.
3. Imaging Analysis
With the advent of deep learning, healthcare providers can now utilize algorithms to analyze medical images with remarkable accuracy. This process can aid radiologists in identifying tumors or fractures that may not be immediately visible.
The Future of Medical Datasets and Machine Learning
The landscape of machine learning in healthcare is continually evolving, fueled by advancements in technology and increasing availability of medical datasets for machine learning. Several trends are shaping the future, including:
- Interoperability: Enhanced data-sharing capabilities will foster collaboration between various healthcare entities, leading to a more holistic view of patient health.
- Real-time Analytics: As wearables and mobile health applications gain traction, real-time data will allow for immediate intervention, improving patient outcomes.
- Ethical AI: There will be a stronger emphasis on ethical practices in the use of AI and machine learning, ensuring that algorithms do not perpetuate biases or inequalities in healthcare.
Conclusion: Embracing the Future of Healthcare
The integration of medical datasets for machine learning in the healthcare sector heralds a new era of patient care and operational effectiveness. By addressing existing challenges and leveraging available resources wisely, healthcare professionals and organizations can significantly enhance medical practices, ultimately leading to improved patient outcomes.
In conclusion, the role of medical datasets in machine learning signifies a crucial step forward in the optimization and personalization of healthcare. As we move into the future, harnessing the power of these datasets will not only facilitate groundbreaking discoveries but also ensure that healthcare services remain focused on providing the best possible outcomes for patients around the globe.
medical dataset for machine learning